AI in Healthcare and the transformation it brings
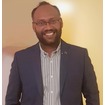
The rise of AI in recent years has been phenomenal and the latest AI-based products and services that have been released to the masses have only helped gain wider adoption. Despite concerns about the downsides, challenges, and risks associated with this next-gen technology, it has managed to hit the right notes for almost anyone who has experienced it.
Any technology that can change humanity for good, is worth investing more time in research and improving it to a stage where it can be adopted on a wider scale. AI stands as a prime example, already making significant strides in healthcare systems and beyond.
In healthcare, AI-driven solutions have revolutionized patient care and management. Here are some notable examples:
- Luscii App: This app remotely monitors and guides patients to avoid unnecessary visits to a medical facility thereby expediting care for those in need. It is integrated with industry-standard APIs such as FHIR (Fast Healthcare Interoperability Resources – a global industry standard for exchanging healthcare data between systems) and EHR (Electronic Health Records) to streamline healthcare processes.
- Moderna’s mRNA vaccine: Modena’s mRNA-based COVID-19 vaccine was developed using the platforms they had envisaged and built pre-COVID which was able to develop vaccines not just faster but with higher potency. This platform is put in use to generate many mRNAs of which the COVID-19 vaccine is just one example.
- Computer Vision AI: AI algorithms are used to scan and analyze medical images and data generated in various medical fields such as dermatology, radiology, or pathology for accurate diagnosis of several diseases.
- Automation: AI helps to automate repetitive tasks with a high degree of precision. AI can automate probably about 80 to 90% of activities/tasks that are predictable and mundane leaving only the remaining 10% to 20% of the same to the physicians, for example, sample inspection. This helps offload the burden of the physicians so that they can use their time to focus on solving challenges that require human intelligence, experience, and expertise to diagnose critical and complicated cases.
Healthcare is one field where AI can never replace humans (doctors, nurses, care workers, etc.), but as you can notice above it can positively and significantly augment human efforts. However, introducing this new technology also brings along challenges associated with it. Below are a few examples of the same.
- Attacks or tampering of AI applications/algorithms to compromise life-critical applications and devices in healthcare facilities severely hampering their operations and causing serious/fatal damages to patients and facilities.
- Cybersecurity attacks such as data breaches of critical medical and financial information, ransomware, and malware attacks.
- Securing IoT/OT-based medical devices and supporting infrastructure.
- Robust IT systems and infrastructure that support the IoMT and supporting applications ecosystem.
- Scalability and Flexibility from its Data Lakes and AI-based Analytics engines.
To address these challenges, IT enterprises and healthcare organizations need to focus on the below areas to build and deploy solutions.
- AI-based Applications and use cases for the Healthcare sector: AI-based applications such as Ai Cure, FitBit, Garmin etc. play a pivotal role in enhancing various facets of patient care and organizational operations. These applications are instrumental in improving diagnostics, treatment planning, and overall patient outcomes by leveraging advanced algorithms and data analytics. By analyzing vast datasets, AI-powered systems can offer valuable insights to healthcare providers, enabling them to make more informed decisions and deliver personalized care.
- Security & Compliance: Healthcare organizations must prioritize robust cybersecurity measures to safeguard sensitive patient information. This involves adhering to strict regulatory standards such as HIPAA and implementing comprehensive security protocols to prevent data breaches and unauthorized access.
- Data Analytics and Inter-operability: Data analytics and interoperability is crucial for ensuring seamless communication and collaboration across different healthcare systems and platforms. By consolidating diverse data sources and enabling interoperability, healthcare organizations can access a comprehensive view of patient information, leading to more coordinated and effective care delivery.
- IT Performance through Automation and AIOps: Automation of IT processes and the adoption of AIOps (Artificial Intelligence for IT Operations) can significantly enhance the performance and reliability of healthcare IT infrastructure. By automating routine tasks and leveraging AI-driven insights for proactive monitoring and maintenance, organizations can optimize their IT systems to support uninterrupted healthcare operations and ensure high levels of efficiency and service reliability.
Additionally, it is important to cover all the areas specific to the solution holistically by following best practices right from the design to the delivery phase. Further fine-tuning should be conducted based on the experiential knowledge gained during this process before making it production-ready.
Let us consider a medical scenario how AI can be leveraged here and what challenges we can expect. Here we are taking a scenario of a woman’s journey through pregnancy wherein it is essential to track, as it could help prevent any untowardly incident if prompt medical attention is sought. To deliver a solution for this scenario the typical workloads and the process involved are:
- IoMT devices that would track, measure, and send the essential pregnancy health parameters-related data in real time or periodically.
- The device data is then received into IoMT FHIR Connector for Azure, where it is normalized, grouped, transformed, and persisted into the Azure API for FHIR.
- The data that is persisted in the Azure FHIR service as FHIR observations is exported to the Azure Data Lake Gen2 using the Bulk Export feature.
- The data would further be cleaned and transformed using Azure Databricks. Scalable machine learning (ML) techniques can be used to derive deeper insights from this data using Python, R, or Scala, with inbuilt notebook experiences in Azure Databricks.
- Azure Databricks integration with Azure ML through MLflow allows for rapid model experimentation.
- Azure ML service can then be used to publish the trained model onto an Azure Container Instance (ACI) or AKS, which can be used to predict any issues in the pregnancy based on the ingested data.
To address these challenges effectively, below listed aspects and solutions must prioritized.
- Security: Security solutions such as Microsoft Defender for Cloud, Defender for IoT, and Microsoft Sentinel can be leveraged to protect the workloads and data on the cloud, IoMT, and other endpoint devices, and the data shared between these applications and devices in transit and storage.
- Compliance: Azure’s healthcare industry certifications, including HIPAA and HITRUST, along with Azure Health Data Services support ensure compliance with industry standards. It is important to note that although the platform supports this capability, at the data layer it is the customer’s responsibility to ensure their application and data both ensure and manage the data privacy of the PHI (Protected Health Information).
- Data Interoperability: Utilizing FHIR service in the solution enables the possibility of interoperability with other disparate systems such as the EHR (Electronic Health Record) systems, thereby allowing health data querying and exchange across multiple disparate systems. Additionally, utilizing FHIR service's data conversion and PHI de-identification capabilities, a central HIPAA-compliant secondary dataset can be created which can be used for analytics and research.
- Performance: AI-driven services must be inherently performant to deliver timely and accurate insights for healthcare decision-making. Factoring all these aspects will help deliver a secure, compliant, inter-operable, flexible, scalable, and performant cloud-based solution that will aid in unifying the scattered PHI data to be able to deliver analytics and insights via ML and AI to solve and speed up various healthcare challenges.
The remarkable advancements in AI technology offer promising solutions for enhancing healthcare outcomes. While its potential is vast, challenges persist. Addressing challenges such as security, compliance, interoperability, and performance is essential for realizing its full benefits. With careful consideration and implementation, AI stands poised to revolutionize patient care and medical practices, ushering in a future of improved health outcomes and enhanced well-being for all.
Microland empowers customers to leverage the transformative potential of Generative AI through its Managed Services and Managed Hosting offerings, providing end-to-end support from solution design to deployment while prioritizing security, compliance, and operational excellence. We provide a secure and compliant multi-tenanted, multi-region platform on Azure to enable our clients to rapidly adopt the powerful capabilities of Gen AI while being assured that they are complying with data residency policies. With the platform secured with multiple controls and, we deliver a solution that complies with defense-in-depth and zero trust principles as well as industry standards like ISO27001 and NCSC guidelines.
The platform is managed from ISO 27001, and ISO 27701 certified delivery centres. In addition, Our UK delivery centres are CES+ certified and operated by Security Cleared staff.