How Generative AI is Accelerating the Transformation of Enterprise Networks
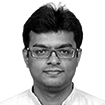
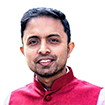
The need for rapid adaptability to change and uninterrupted and superior digital experiences for their end customers is a top priority for business leaders in global digital enterprises. To keep up with the dynamic needs of enterprises from their digital infrastructure, business leaders are looking to transform enterprise networks for greater speed, reliability, and availability.
Owing to its self-healing nature, Network Automation and AIOps are one of the top 3 network transformation initiatives that enterprises have undertaken in the last few years to boost productivity and enhance business growth.
Network Automation has already shown results by accelerating network transformation projects with zero-touch and zero-error provisioning, deployments, and testing, and in network operations, reducing network incidents and performance degradation issues through proactively reducing business downtimes leading to enhanced reliability, availability, and performance of enterprise networks, and reduced operational costs which at a business level is translating to unfaltering network experience and improved productivity for business users and end clients.
In 2021, the global market of network automation was around USD 14.56 Billion. By 2030, it is estimated to grow to be a USD 72.4 Billion market at a CAGR of 19.5%. Estimation of such tremendous growth in the network automation market shows the enhancing interest and demand of this technology.
The increased adoption of Network Automation is due to the increased technical and business complexity of enterprise networks, the adoption of the Internet of Things and next-gen networking technologies like software-defined networks, Cloud-native Network Functions (CNFs), and 5G technology, integration of networking and security technologies and the increased alignment and optimization of enterprise networks with business objectives and growth goals.
Since the launch of ChatGPT from OpenAI in 2022, GenAI has become a powerful and versatile AI platform that is helping enterprises transform various aspects of their operations. It has also had a significant impact on network transformation and operations. Enterprises have identified GenAI use cases in automating network design, configurations, management, and optimization, and generating insights from infrastructure analytics.
Generative Use Cases in Network Transformation
Generative AI is being leveraged by Network Architects and Engineers to analyze network requirements and generate optimal network topology and device settings followed by designing and configuring network devices.
- Network design and planning: GenAI can help network architects assess and perform analytics on network performance metrics like bandwidth, latency, reliability, availability, etc., to design and plan optimal network architectures, topologies, and configurations that meet the business requirements and objectives. GenAI can also perform network simulations and validations to ensure the feasibility and efficiency of the network design. It is also leveraged to validate the network design and configuration against best practices and industry standards
- Network deployment and provisioning: GenAI can automate the network deployment and provisioning process, by orchestrating and executing the network configuration commands, policies, and rules across different network devices and domains. GenAI can also verify and optimize the network deployment and provisioning outcomes, by detecting and resolving any errors, conflicts, or inconsistencies.
This helps Network Architects and Engineers by
- Reduce the time taken in network assessments and topology design
- Optimize network design to address the requirements
- reduce human errors in assessment, design, and configurations
- faster time to market with automated deployments
- reduced network downtime and costs during the transformation
Generative Use Cases in Network Operations
In Network Operations, Generative AI is being integrated with Network Automation and AIOps tools to optimize network configurations and policies and accelerate incident RCA (Root Cause Analysis) and resolution through alert clustering, troubleshooting, and documentation in human-understandable language for knowledge management.
Generative AI is also being leveraged to analyze vast amounts of network data such as traffic, utilization, availability, and device health being generated in real-time and provide real-time analytics and insights based on historical performance trends. It can also identify recurring network issues such as congestions, bottlenecks, and other anomalies to provide performance-optimizing recommendations for automated load balancing, routing, and bandwidth allocation in software-defined networks.
- Network monitoring and management: GenAI is being leveraged to monitor and manage the network availability, performance, quality, and health across multiple domains, layers, and devices by analyzing the real-time data collated from multiple sources and monitoring tools and logs, identifying, and diagnosing network incidents and problems. It is also being leveraged for predictive issue resolution by using generative models to analyze network data and traffic and anticipate the network performance and behaviors.
- Network optimization and tuning: GenAI is also helping in tuning and optimizing the network configurations, policies, and resources to align with the business objectives and customer expectations, by using generative models to simulate network data and traffic, predict future network needs based on historical trends and tune network configurations and policies to improve the performance and availability. Integrated with network automation tools, GenAI algorithms automatically optimize the configurations and network parameters such as bandwidth allocation, load balancing, routing, etc., based on the network performance conditions, user experience, and business requirements.
- Network Security and Compliance: GenAI and Machine Learning algorithms are being employed to perform behavior analysis and detect and classify network security anomalies and threats such as unauthorized access, data breaches, malware attacks, etc., to protect network assets and data. It also helps network administrators optimize network security policies and configurations and conduct audits to comply with regulations and compliance requirements.
- Network analytics and insights: Generative AI is being leveraged to provide insights and recommendations from network analytics on parameters such as network performance, utilization, availability, reliability, and customer satisfaction, using real-time dashboards and reports with visualizations. GenAI is also providing recommendations and feedback to network administrators, such as network best practices, opportunities for continuous improvement initiatives, and cost optimization.
- Network innovation and value creation: GenAI is also helping network administrators and architects to create value by continuously innovating and improving based on the network data and insights, by using generative models to create and tune network performance scenarios, and analytical models to extract network insights.
The above GenAI use cases in Network operations can,
- Significantly reduce network downtime further by proactively detecting and fixing network issues
- Increased network performance and efficiency by optimizing network resources and utilization
- Lowered operational costs and complexity by automating and simplifying network troubleshooting processes, reducing detection and resolution times.
- Increased network performance and efficiency by optimizing network resources and utilization, enhanced network agility and scalability by enabling network flexibility and adaptability
- Increased network visibility and insight by providing comprehensive and contextual information about network health and performance
- Improved network security and resilience by preventing and mitigating network incidents and threats
- Enhanced network compliance and governance by ensuring alignment with security standards and best practices
- Reduced risks by minimizing human errors
- Improved business-user experience and productivity
Challenges of GenAI in Network Transformation and Operations
These GenAI use cases have enhanced Network Automation and AIOps tools capabilities to move towards completely self-healing and autonomous networks. However, since GenAI is still in its nascent stages, it is not without its challenges. Below are some of the challenges that Network Managers, Architects, Administrators, and Engineers face while leveraging GenAI in network transformation and operations.
- Data quality and availability: GenAI relies on large and diverse datasets to generate high-quality and relevant insights and recommendations. However, enterprise networks may not have enough data or the right kind of data to train and fine-tune GenAI models. Moreover, data privacy and security issues may limit the access and sharing of data across different entities and stakeholders. Data insufficiency is a problem, especially for Medium and Small enterprises with a small network estate.
- Model explainability and trustworthiness: GenAI models are often complex and opaque for Network Administrators and Engineers to understand, making it hard to understand how they generate their outputs and whether they are reliable and consistent. This may reduce the trust and confidence of network operators and customers, who may not be willing to accept or use GenAI-generated solutions without proper explanation and validation.
GenAI is a revolutionary technology that is transforming every industry across the globe and has shown similar promise in enterprise network transformation and management. In the early use cases identified above, we are already seeing enterprise networks being managed more effectively and efficiently, leading to reduced costs, improved security, and user experience.
Despite GenAI showing a positive impact in the early use cases and significant potential in the future in enhancing Automation and AIOps capabilities in Network Transformation and Operations, it also poses significant challenges and risks that need to be carefully considered and addressed. Enterprise networks should adopt a responsible and ethical approach to leveraging GenAI, by ensuring data quality and availability, model explainability and trustworthiness, human-AI collaboration and governance, and ethical and social implications.
Since it is in its nascent stages, it will take some time to become capable, reliable, and secure to make networks self-healing and autonomous, and at the same time affordable to most enterprises, especially medium and small enterprises.